취미가 좋다
CyCADA: Cycle-Consistent Adversarial Domain Adaptation 논문 정리 본문
CyCADA: Cycle-Consistent Adversarial Domain Adaptation 논문 정리
benlee73 2021. 5. 4. 14:39
CyCADA: Cycle-Consistent Adversarial Domain Adaptation
Judy Hoffman, Eric Tzeng, Taesung Park, Jun-Yan Zhu, Phillip Isola, Kate Saenko, Alexei A. Efros, Trevor Darrell
이전 ADDA 에서 Cycle GAN을 추가한 논문이다.
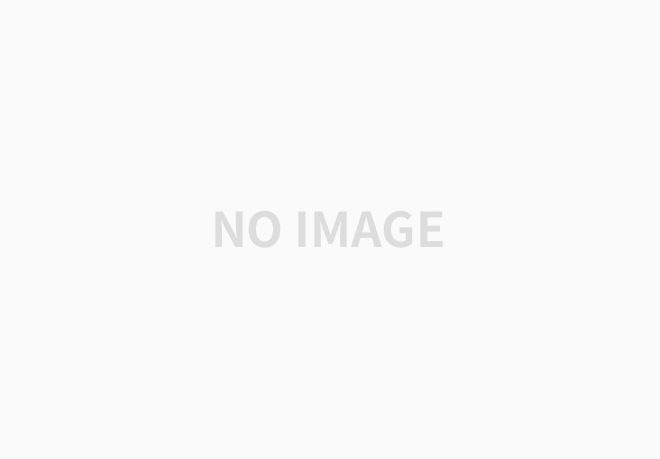
G(S→T) 는 source image로부터 Target image를 만드는 generator이고, G(T→S)는 target image로부터 source image를 만드는 generator이다.
Generator가 2개이므로, 입출력을 구별하는 Discrimitor도 2개이다.
G(S→T)와 G(T→S)를 이어서 적용하여 나온 결과 값과 원본 이미지와의 Cycle loss를 학습에 반영한다.
2 stage로 학습이 진행된다.
우선 Cycle GAN으로 source image를 target image 스타일로 변환한다.
이후 변환된 source image와 target image를 ADDA 방식으로 학습한다.
결국 pixel level과 feature level 두 곳에서 도메인 격차를 줄이는 셈이다.
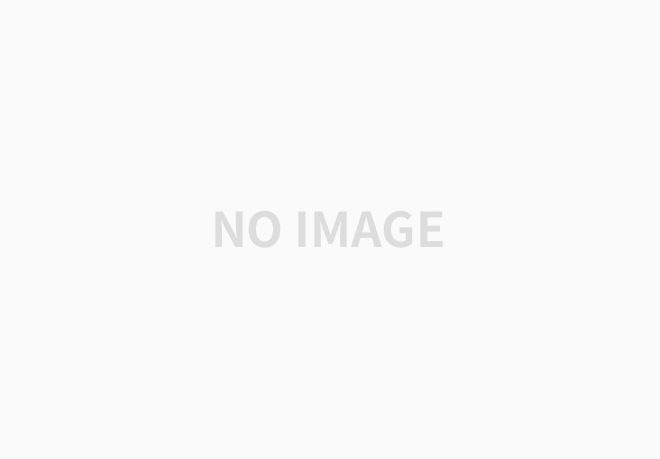
이전에 작성한 논문의 ADDA처럼 feature level에서 adversarial loss를 적용할 뿐만 아니라, pixel level의 cycle loss도 적용하여 발전시켰다.
그래서 위의 표를 보면 ADDA 보다 성능이 향상된 것을 확인할 수 있다.
아래와 같이 이미지 변환도 잘 이루어지는 것을 확인할 수 있다.
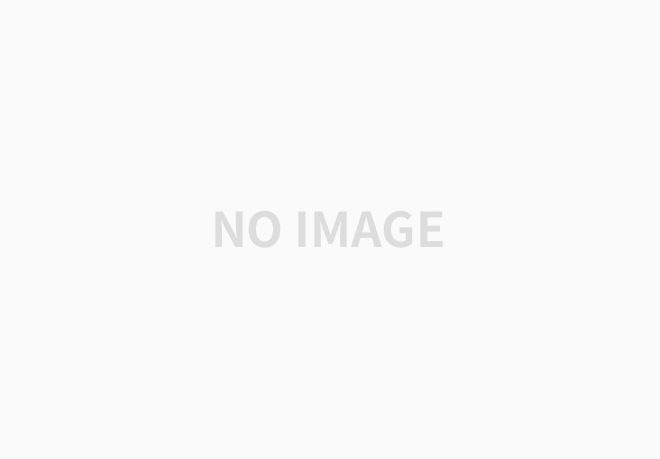
'논문 > Domain Adaptation & Generalization' 카테고리의 다른 글
Learning to Learn Single Domain Generalization 논문 정리 (0) | 2021.05.11 |
---|---|
Do Adversarially Robust ImageNet Models Transfer Better? (0) | 2021.05.04 |
Bidirectional Learning for Domain Adaptation of Semantic Segmentation 논문 정리 (0) | 2021.05.04 |
Adversarial Discriminative Domain Adaptation 논문 정리 (0) | 2021.05.04 |
Unsupervised domain Adaptation by Backpropagation 논문 정리 (0) | 2021.05.04 |